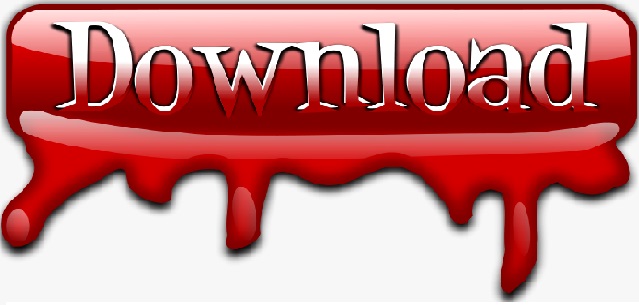

The most obvious is reducing latency between an event occurring and taking an action driven by it, whether automatic or via analytics presented to a human. With a platform such as Spark Streaming we have a framework that natively supports processing both within-batch and across-batch (windowing).īy taking a stream processing approach we can benefit in several ways.

Spark url extractor python code#
Whilst intra-day ETL and frequent batch executions have brought latencies down, they are still independent executions with optional bespoke code in place to handle intra-batch accumulations. Processing unbounded data sets, or "stream processing", is a new way of looking at what has always been done as batch in the past. You can read more in the excellent Streaming Programming Guide. It does this by breaking it up into microbatches, and supporting windowing capabilities for processing across multiple batches. Spark Streaming provides a way of processing "unbounded" data - commonly referred to as "streaming" data. This is one of several libraries that the Spark platform provides (others include Spark SQL, Spark MLlib, and Spark GraphX). In this article I am going to look at Spark Streaming. The processing that I wrote was very much batch-focussed read a set of files from block storage ('disk'), process and enrich the data, and write it back to block storage. This was in the context of replatforming an existing Oracle-based ETL and datawarehouse solution onto cheaper and more elastic alternatives.
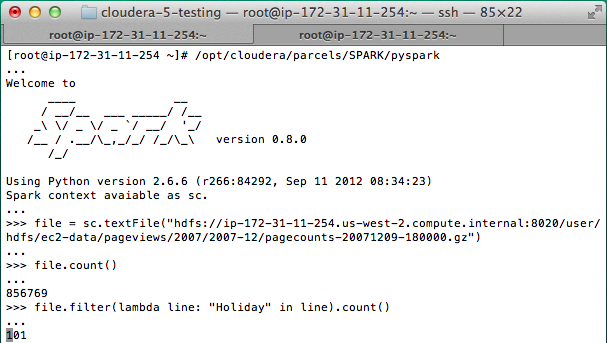
Last month I wrote a series of articles in which I looked at the use of Spark for performing data transformation and manipulation.
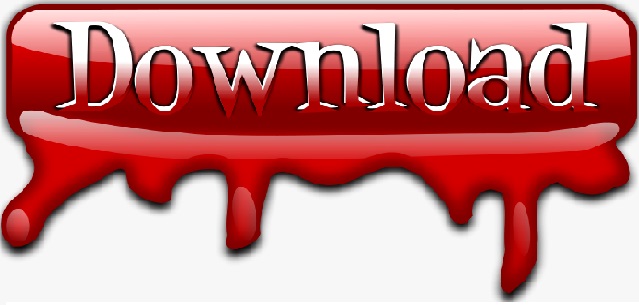